Potential of AKT2 expression as a predictor of lymph-node metastasis in invasive breast carcinoma of no special type
Article information
Abstract
Background
Invasive breast carcinoma of no special type (IBC-NST) is the most common type of breast cancer and mainly causes regional lymph-node metastasis (LNM). We investigated the potential for AKT2 expression as a predictive biomarker for LNM in IBC-NST.
Methods
Forty-eight paraffin blocks containing IBC-NST primary tumors were divided into two groups based on presence or absence of LNM. Age, tumor grade, tumor size, lymphovascular invasion (LVI), and AKT expression were assessed. AKT2 expression was assessed based on immunohistochemical staining, while other data were collected from archives.
Results
Multiple logistic regression results showed that AKT2 expression and LVI were significantly associated with LNM (odds ratio [OR], 5.32; 95% confidence interval [CI], 1.42 to 19.93 and OR, 4.46; 95% CI, 1.17 to 16.97, respectively). AKT2 expression was able to discriminate against LNM (area under the receiver operating characteristic, 0.799 ± 0.063; 95% CI, 0.676 to 0.921) at an H-score cutoff of 104.62 (83.3% sensitivity, 62.5% specificity).
Conclusions
AKT2 expression has potential as a predictor of LNM in IBC-NST. The H-score cutoff for AKT2 expression can be used as a classification guide in future studies.
Breast cancer is the most common and deadly type of cancer in women, accounting for 25% of all cancers in women. The 2018 global breast cancer incidence reached 2.09 million new cases [1]. Breast cancer results from a tumor that arises from uncontrolled proliferation of breast tissue. To improve identification, histological breast cancer classification is divided into two types based on the relationship to the basement membrane: non-invasive breast carcinoma (nIBC) and invasive breast carcinoma (IBC) [2]. IBC has faster progression, many subtypes, and is more common than nIBC. Among the IBC subtypes, 70% are invasive breast carcinoma of no special type (IBC-NST) [2]. Higher breast cancer grades indicate worse prognosis, and the likelihood of metastasis is greater in carcinomas with poor grading, such as with IBC-NST. Metastasis is a progression stage wherein cancer cells spread and invade other organs [3]. Metastasis occurs by forming new cancer cell colonies in other healthy body tissues, further reducing patient prognosis [3]. In IBC-NST, the most common body tissue for metastases is axillary lymph nodes, hereinafter referred to as lymph-node metastasis (LNM).
Various efforts to improve LNM prediction have been made for tertiary prevention in breast cancer patients, one of which is identification of specific IBC-NST biomarkers [4]. Many studies have investigated potential IBC-NST biomarkers, including AKT, which is a protein involved in IBC-NST progression. AKT is located in the cell membrane, and cytoplasm activates various downstream protein substrates that instigate cancer progression [5]. However, according to a systematic review by Yang et al. [6], many studies have produced inconsistent findings regarding the role of AKT in breast cancer. These discrepancies might be because AKT is thought to have three isoforms— AKT1, AKT2, and AKT3—each of which has distinct and opposing roles [7]. AKT3 has not been widely studied, but it is suspected to play a greater role in triple-negative breast cancer [7]. AKT1 plays a role in proliferation through upregulation of cyclin D1, but it is thought to inhibit cell migration and invasion through downregulation of β1-integrin and focal adhesion kinase (FAK) [7]. In contrast, AKT2 is thought to induce cell migration and metastasis through induction of vimentin and F-actin [7]. Responding to this problem, we see the potential for AKT2 as a predictor of LNM in IBC-NST. AKT2 is one of the proteins involved in cancer invasion pathways and epithelial mesenchymal transition (EMT), both of which affect patient prognosis [8]. However, there are no studies into AKT2 as a predictor of LNM in IBC-NST. Therefore, this study investigates the role of AKT2 expression as a potential predictor of LNM in IBC-NST and assesses its potential as a predictive biomarker of IBC-NST.
MATERIALS AND METHODS
Study design and data collection
This is a cross-sectional study that was conducted in the Anatomical Pathology Laboratory of the Faculty of Medicine at the University of Indonesia from December 2019 to December 2020. The study conforms with The Code of Ethics of the World Medical Association (Declaration of Helsinki) [9]. Data retrieved from the 2019 and 2020 departmental archives were patient age, tumor subtype (limited to IBC-NST), tumor grade (based on Nottingham Grading System [10]), tumor size, lymphovascular invasion (LVI), and LNM. AKT2 expression data were obtained through quantification of immunohistochemistry (IHC) staining results.
Samples
The samples evaluated in this study were primary tumor paraffin blocks from breast mastectomy in Asian females that had been diagnosed histopathologically with IBC-NST, either with or without metastasis into adjacent lymph nodes. Samples having an additional histopathological diagnosis other than IBC-NST (e.g., invasive lobular carcinoma, medullary carcinoma, papillary carcinoma), systemic comorbidities (hypertension, diabetes mellitus, etc.), or damaged paraffin blocks (e.g., paraffin blocks with tumor masses cut or eaten by animals, etc.) were excluded.
Samples were classified according to presence or absence of LNM. The minimum sample size was calculated with alpha = 5%, confidence interval (CI) = 95%, and power = 80%. Consequently, a minimum of 23 samples was required for each group. We obtained 24 samples for the metastatic lymph node group and 24 samples for the non-metastatic lymph node group. To avoid bias, grouping data were accessed by a single researcher (K.K.), and the other researchers were blind to patient groupings until the research process was complete.
Slide preparation and IHC staining
Slide preparation was performed by cutting the tissue in a paraffin block using a microtome to a thickness of 3–5 μm. Next, the sample was heated on a slide warmer (30–60 minutes) at 58°C, deparaffinized using stratified xylol (Merck, Jakarta, Indonesia) for 5 minutes, rehydrated in alcohol (Merck) for 5 minutes, and rinsed with water for 5 minutes. Each slide was pre-treated with heat-induced retrieval antigen using 0.1 M NaOH citrate buffer pH 7.0 (Brataco Inc., Jakarta, Indonesia) in an autoclave at 121°C for 15 minutes and then washed in phosphate buffered saline (PBS) pH 7.4 (Brataco Inc.) for 5 minutes. Blocking was performed using hydrogen peroxide (Brataco Inc.) in 3% v/v methanol (Brataco Inc.) for 30 minutes at room temperature. Next, it was washed under running water for 5 minutes, followed by nonspecific protein blocking with universal Background Sniper (Abcam, Jakarta, Indonesia) for 15 minutes. After the blocking process, the slides were incubated for 1 hour with anti- AKT2 antibody (Abcam) at a 1:100 dilution and then washed in PBS for 5 minutes. Subsequently, the slides were incubated with biotinylated secondary antibody (Abcam) for 30 minutes and washed in PBS for 5 minutes. Diaminobenzidine tetrahydrochloride (Abcam) was dropped onto a slide and counterstained with Lillie-Mayer's hematoxylin (Abcam) for 2 minutes. Subsequently, the slides were immersed in lithium carbonate (Merck) for 2 minutes followed by graded alcohol dehydration (5 minutes) and graded xylol clearing (5 minutes). Finally, the sections were covered with a liquid cover, which is an aqueous mounting media. The stained sections were subsequently examined for AKT2 expression. Negative and positive controls were included for each staining. The negative control was established by eliminating the primary antibody administration step.
Quantification of AKT2 expression
Immunohistochemical staining assessment was performed by two experienced researchers (P.R. and K.A.B.). Each preparation was observed using a light microscope at 400x magnification and was documented using Leica LAZ EZ software (Jena, Germany) and a camera that was integrated with a Leica DM750 microscope. AKT2 expression was assessed in at least 500 tumor cells from 5 high-power visual fields (×400) that were chosen randomly. Each region was represented by a minimum of 100 tumor cells. AKT2 positivity was represented by brown staining of the tumor cell membrane and cytoplasm.
Staining intensity was categorized into no staining (0), low positive (1+), positive (2+), and high positive (3+) based on the intensity of the brown color observed in each view field using the cell counter function in ImageJ [11]. The H-score was calculated to quantify AKT2 expression and was based on the following formula [12]:
(1)
Two observers (P.R. and K.A.B.) independently calculated the H-scores for all samples. To avoid bias, the results of the previously assessed calculations were reported to the statistician (E.W.) until the entire sample was assessed. The mean H-score of the two observers was used for statistical analyses.
Statistical analyses
Data were entered into a master table using Microsoft Excel 2013 (Microsoft Corp., Redmond, WA, USA), and the tabulated data were analyzed using the SPSS ver. 20 (IBM Corp., Armonk, NY, USA) and visualized using GraphPad Prism 8 (GraphPad Software, Inc., La Jolla, CA, USA). Variability in H-score between the two observers was compared with the intraclass correlation coefficient (ICC) to assess data reliability. The ICC model used a two-way mixed average measurement with absolute agreement. ICC values were grouped according to 95% confident intervals of the ICC estimate: poor, < 0.5; moderate, 0.5–0.75; good, 0.75–0.9; and excellent, > 0.90.
H-scores of the two observers were averaged and grouped into high or low groups using the median H-score as the cutoff (median split approach) [13,14]. This grouping describes AKT2 expression in each sample. A bivariate analysis was performed to compare age (< 50 years or ≥ 50 years), tumor grade (high or low), tumor size (≤ 5 cm or > 5 cm), LVI (yes or no), and AKT2 expression (high or low) against LNM (yes or no). All variables that have a p < .2 in bivariate analysis will be included in multivariate multiple logistic regression models using the backward logistic regression method. The discrimination capacity of the model was calculated from the area under the receiver operating characteristic (AUROC) curve. A p-value less than .05 was considered statistically significant.
The receiver operating characteristic (ROC) curve for the AKT2 H-score was analyzed, for which we included H-score as a continuous variable. ROC was determined according to the area under the curve (AUC) that was grouped as follows: 0.5, no discrimination; 0.7–0.8, acceptable; 0.8–0.9, excellent; and > 0.9, outstanding. The AKT2 expression cutoff value was assessed from the ROC curve based on the highest Youden Index [15] and the lowest K-Index [16]. The Youden Index uses the maximum vertical distance of the ROC curve from the (x, y) point on the diagonal (chance) line.
RESULTS
IHC staining and H-score reliability
All 48 samples underwent immunohistochemical staining for AKT2 expression. Representative immunohistochemical staining results are shown in Fig. 1A–D. Each image represents a sample of tumor cells with different staining groups: high positive (A), positive (B), low positive (C), and no staining (D). The images include several visual fields from the same slide. These photos also show that, in one slide, sometimes even in one visual field, several cells can be found with different intensities. AKT2 expression can be observed in the cell membrane and cytoplasm. The intensity of brown color in these areas was quantified into an H-score for further assessment.
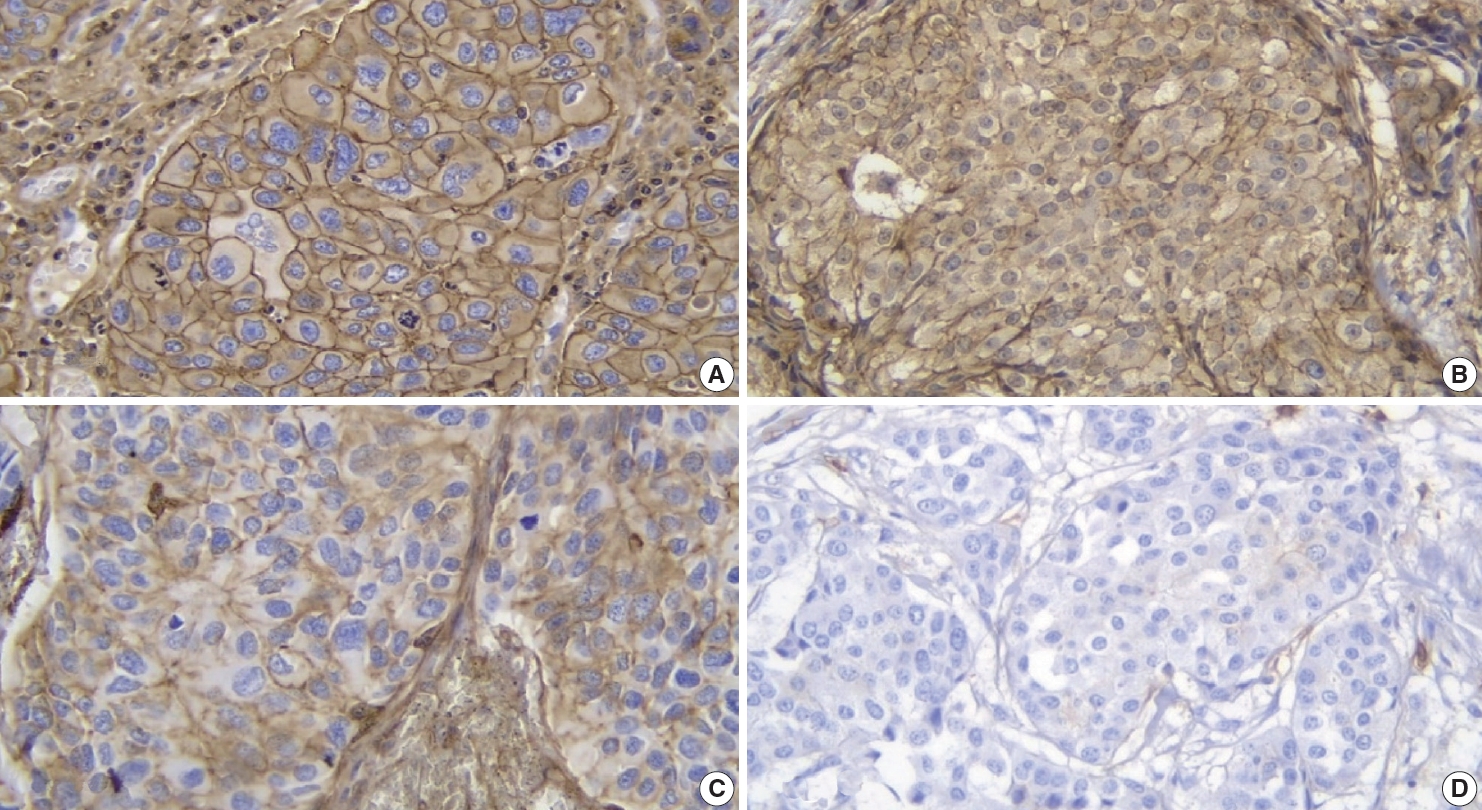
Immunohistochemical staining for AKT2 expression in IBC-NST tumor cells: (A) high positive, (B) positive (C) low positive, and (D) no staining. IBC-NST, invasive breast carcinoma of no special type.
Two observers (P.R. and K.A.B.) assessed all 48 samples independently. The distribution of H-scores within each sample can be seen in Fig. 2. Reliability between any two measurements was determined to be moderate. The average measure ICC was 0.726 (95% CI, 0.509 to 0.847; F (47.47) = 3.593; p < .001).
Association between AKT2 expression and LNM
The clinicopathologic characteristics of each sample are presented in Table 1. Each of these variables is a covariate that can have a role in LNM occurrence. Therefore, we considered the role of each variable by conducting bivariate tests to evaluate their relationships with LNM. Bivariate analyses of several variables were performed to assess their association with LNM (Table 2), including patient age (< 50 years or ≥ 50 years), tumor grade (grade III [high] or grade I–II [low]), tumor size (≤ 5 cm or > 5 cm), LVI (yes or no), and AKT2 expression (high or low).
Bivariate analysis results indicated a significant relationship between AKT2 expression and LNM (p = .009). LVI also was significantly associated with LNM (p = .020). There was no significant association between patient age, tumor grade, or tumor size and LNM. Because both AKT2 expression and LVI showed significant associations with LNM, they were included in a multivariate regression model (Table 3).
Both AKT2 expression (odds ratio [OR], 5.32; 95% CI, 1.42 to 19.93) and LVI (OR, 4.46; 95% CI, 1.17 to 16.97) were significantly associated with LNM. Samples with high AKT2 expression were 5.32 times more likely to have metastases than were samples with low AKT2 expression, and samples with LVI were 4.46 times more likely to have metastases than were samples without LVI. We also calculated the metastatic probability based on the following formula [17]:
(2)
, where P(Y) is the probability of LNM, X1 represents AKT2 expression (high, 1; low, 0), and X2 represents LVI (yes, 1; no, 0). From this model, the probability of LNM can be calculated for various conditions (Table 4).
Model goodness-of-fit was adequate (Table 3). The Hosmer- Lemeshow test (p = .999) indicated that LNM numbers were not significantly different from those predicted by the model, and model predictions yielded satisfactory goodness-of-fit. Moreover, the difference between the overall model and a constant-only model was statistically significant (p = .001), indicating that the predictors reliably distinguished between LNM and non-LNM cases. The AUROC value for this model was 0.78, which indicates excellent discriminatory ability.
ROCs of AKT2 expression
The ability of AKT2 expression to discriminate against lymphnode metastases was assessed through ROC curve analysis (Fig.3). The AUC was 0.799 ± 0.063 (95% CI, 0.676 to 0.921). This area (approximately 0.8) showed excellent accuracy. The optimal cutoff point for AKT2 expression was 104.62 (H-score), which yielded the highest Youden Index (0.458) and lowest Kindex (0.41) among all cutoffs. This H-score has 83.3% sensitivity and 62.5% specificity.
DISCUSSION
Biomarkers are objectively measured characteristics that indicate normal biological processes, pathological processes, pharmacological responses, or therapeutic interventions as proteins that function under specific conditions. AKT2, one of the AKT isoforms, has a role in cancer invasion and metastasis. Thus, AKT2 identification is important for assessing cancer cell characteristics that cause LNM [18]. There are various techniques for identifying AKT2 expression in cells, including polymerase chain reaction (PCR), fluorescence in situ hybridization (FISH), and other molecular methods. In this study, we used a relatively convenient and affordable technique, IHC staining, to identify AKT2. IHC is a common method to identify biomarkers using selective antibodies to bind to specific proteins in tumor cells. While other more advanced molecular methods, such as PCR and FISH, are increasingly used in clinical practice, they can be less practical than IHC [19].
Specific antibodies bind to AKT2 in the cell membrane and cytoplasm and, to visualize them, diaminobenzidine tetrahydrochloride was used as a chromogen to emit a brown color (Fig. 1). The higher was the intensity of the brown color, the higher was the expression of AKT2 in cells. Also, because AKT2 is located mainly in the cell membrane and cytoplasm, brown color can be observed in those areas of the cell [5]. This is consistent with Trigka et al. [20], where 94.14% of their samples had immunoreactivity against AKT2 staining in cytoplasm and cell membranes. Research by Malik et al. [21] showed that the staining was localized to the membrane where AKT2 was observed to be active. These findings strengthen the value of IHC as a practical method for AKT2 detection.
To confirm AKT2 expression as a predictor of LNM, several known confounding factors had to be controlled [22], including patient race, additional histopathological diagnoses, tumor subtypes, and multiple comorbidities. We eliminated these factors through application of inclusion and exclusion criteria. Several other confounding factors, including age, tumor grade, tumor size, and LVI, were included in the model, but their association effect with LNM was controlled through bivariate and regression analyses. Among these confounding factors, only LVI has a significant relationship with LNM. After regression analysis to analyze the relationship between AKT2 expression and LNM, LVI was added as a covariate but did not change the effect of AKT2 expression on LNM. This suggests AKT2 expression as an independent predictor of LNM.
The association between AKT2 expression and LNM in IBC-NST is interesting. In the logistic regression model, AKT2 expression had a very high OR (5.32; 95% CI, 1.42 to 19.93), meaning that primary tumor samples with high AKT2 expression were 5.32 times more likely to have LNM than samples with low AKT2 expression. This relationship is due to underlying molecular mechanisms–as previously explained, AKT2 has a promigratory role in cell migration, invasion, and metastasis. AKT2, but not AKT1 or AKT3, enhances integrin β1-mediated attachment and invasion through collagen IV, which plays an important role in cell invasion and migration [23]. Furthermore, AKT2 directly interacts with PKCζ, which activates adhesion-associated β1-integrin and the actin-polymerizing LIMK/Cofilin axis after epidermal growth factor stimulation [23]. Additionally, AKT2 stimulation by mammalian target of rapamycin in Ser473 causes glycogen synthase kinase-3β degradation, which also triggers EMT [23]. This is consistent with Ye et al. [24], who found that AKT2 promoted breast cancer cell growth after G proteincoupled receptor stimulation. Chen et al. [25] also demonstrated an important role for AKT, finding that cPLA2α mediates EMT via transforming growth factor β activation in the phosphoinositide 3-kinase/AKT pathway. All of these results support the role of AKT2 in inducing LNM.
We also found an association between LVI and LNM (OR, 4.46; 95% CI, 1.17 to 16.97), indicating that primary tumor samples with LVI were 4.46 times more likely to show lymphnode metastases than samples without LVI. The molecular mechanisms underlying LVI are unclear. In their review, Kariri et al. [26] state that “understanding the role of cancer cell invasion and migration in the differentiation of LVI-related molecular alterations from these driving tumor cell invasion and migration as an early mechanism associated with malignancy is a ‘difficult’ but ‘beatable’ challenge.” However, several hypothetical mechanisms have been proposed. Ribelles et al. [27] suggested that genetic alterations can increase migratory potential. On the other hand, Melzer et al. [28] suggested that matrix metalloproteinases can disrupt primary tumor stability, inducing migration and, ultimately, LVI. Apart from the underlying molecular mechanisms, several studies have found associations between LVI and LNM. Research by Schoppmann et al. [29] indicates that LVI was associated significantly with a higher risk for developing LNM. In fact, Nathanson et al. [30] showed that LVI can predict systemic metastasis when regional lymph-node metastases are positive. All these findings explain the association between LVI and LNM identified in this study.
On the other hand, we calculated the probability of LNM by including a constant (β) to the model formula (2). In formula (2), the constant (β) is used as a multiplier of the related variable. This also shows that the variable with a greater constant (β) has a more dominant role in determining LNM probability, and vice versa. Therefore, it can be concluded that AKT2 expression is more dominant than LVI in determining LNM probability. Although no previous studies have examined these two components simultaneously, several correlational studies can explain this finding. Research by Wang et al. [31] demonstrated a positive correlation between AKT2 mRNA expression and LNM in breast cancer (r = 0.46, p < .001). Meanwhile, a meta-analysis by Zhang et al. [32] showed a correlation between LVI and lymphnode metastases. However, the correlation was low (r = 0.24; 95% CI, 0.19 to 0.28) [32], indicating that the role of LVI on LNM is less dominant.
The potential for AKT2 as an LNM predictor needs further elucidation. One approach uses LNM discrimination analysis on the ROC curve. The AUC ROC was 0.799 ± 0.063 (95% CI, 0.676 to 0.921), indicating excellent accuracy, in this study, AKT2 expression as a way to discriminate lymph-node metastases was very good. Therefore, we conducted an analysis to estimate an optimal cutoff as a guide for classifying high or low AKT2 expression.
As explained above, this study uses the median H-score to classify AKT2 expression. However, we are aware that use of a marker-specific IHC cutoff assay is important for prediction of therapeutic response [33]. Therefore, we conducted an additional analysis to identify a potential cutoff for AKT2 expression, wherein we estimated an H-score of 104.62. This suggests that, if this cutoff is implemented in an IBC-NST AKT2 expression dataset, an H-score ≥104.62 can be classified as “high AKT2 expression.” On the other hand, values with an H-score < 104.62 can be classified as “low AKT2 expression.” Of course, this cutoff needs to be further explored and refined, but we expect it to help classify AKT2 expression in future studies.
In conclusion, AKT2 expression can be used as a predictor to determine LNM. LVI also can be used as a predictor, although it has a less dominant role. Both play a role in predicting LNM in IBC-NST. Moreover, AKT2 expression can be identified by IHC staining, a practical method, with an H-score cutoff of 104.62 for classifying high and low AKT2 expression. This cutoff can be used in future research regarding AKT2 expression in IBC-NST.
Notes
Ethics Statement
The experimental protocols were approved by the Ethics Committee of the Faculty of Medicine, University of Indonesia (protocol number 20-09- 1169, July 2020). All participants provided written consent, and the study conforms with The Code of Ethics of the World Medical Association (Declaration of Helsinki).
Availability of Data and Material
The datasets generated or analyzed during the study are available from the corresponding author on reasonable request.
Code Availability
Not applicable.
Author Contributions
Conceptualization: PR, EW. Data curation: EW, KAB. Formal analysis: EW. Funding acquisition: PR. Investigation: PR, KAB. Methodology: PR, EW. Software: EW. Validation: PR. Visualization: EW. Writing—original draft: EW. Writing—review & editing: PR, KK. Approval of final manuscript: all authors.
Conflicts of Interest
The authors declare that they have no potential conflicts of interest.
Funding Statement
No funding to declare.
Acknowledgements
We would like to thank all the staff from Department of Anatomical Pathology Faculty of Medicine, Universitas Indonesia.