The application of high-throughput proteomics in cytopathology
Article information
Abstract
High-throughput genomics and transcriptomics are often applied in routine pathology practice to facilitate cancer diagnosis, assess prognosis, and predict response to therapy. However, the proteins rather than nucleic acids are the functional molecules defining the cellular phenotype in health and disease, whereas genomic profiling cannot evaluate processes such as the RNA splicing or posttranslational modifications and gene expression does not necessarily correlate with protein expression. Proteomic applications have recently advanced, overcoming the issue of low depth, inconsistency, and suboptimal accuracy, also enabling the use of minimal patient-derived specimens. This review aims to present the recent evidence regarding the use of high-throughput proteomics in both exfoliative and fine-needle aspiration cytology. Most studies used mass spectrometry, as this is associated with high depth, sensitivity, and specificity, and aimed to complement the traditional cytomorphologic diagnosis, in addition to identify novel cancer biomarkers. Examples of diagnostic dilemmas subjected to proteomic analysis included the evaluation of indeterminate thyroid nodules or prediction of lymph node metastasis from thyroid cancer, also the differentiation between benign and malignant serous effusions, pancreatic cancer from autoimmune pancreatitis, non-neoplastic from malignant biliary strictures, and benign from malignant salivary gland tumors. A few cancer biomarkers—related to diverse cancers involving the breast, thyroid, bladder, lung, serous cavities, salivary glands, and bone marrow—were also discovered. Notably, residual liquid-based cytology samples were suitable for satisfactory and reproducible proteomic analysis. Proteomics could become another routine pathology platform in the near future, potentially by using validated multi-omics protocols.
Since next-generation sequencing (NGS) technologies were introduced, sequencing data output significantly increased and brought unprecedented revolution into cancer genomic profiling [1,2]. In addition, the affordable cost of NGS technologies has made their clinical application feasible, as well as their use in the research setting [2,3]. Comprehensive genetic profiling of tumor samples has driven the construction of The Cancer Genome Atlas (TCGA), comprising enormous genomic landscapes across various cancer types. Notably, NGS-based gene panel tests have put genomic sequencing into routine clinical practice as diagnostic tools enabling precision medicine [4]. In addition to surgical pathology, NGS has been extensively used in the field of cytology, utilizing both exfoliative and fine-needle aspiration (FNA) samples [5-9].
However, the number of transcripts does not necessarily correlate with that of the translated proteins, which are the actual functional molecules defining the cellular phenotype in health and disease. Multiple splicing variants could be formed from each transcript during RNA maturation [10-12], while more than 400 different types of post-translational modifications such as acetylation, phosphorylation, glycosylation, methylation, and peptide cleavage might change the properties of the final protein product [12-14]. Furthermore, it may be difficult to define which mutations are the driver and passenger ones while analyzing nucleic acids. All these may limit our understanding of the complexity of cancer and our quest for optimal diagnostic, prognostic, and therapeutic biomarkers, especially when counting solely on data derived from genomics and/or transcriptomics [15]. Thus, the integration of multi-omic approaches, including genomics, epigenomics, transcriptomics, proteomics, and/or metabolomics, could combine the strengths of each high-throughput application, enhancing cancer diagnosis, prognosis, and therapy [16,17].
In the past, classic analytical methods to detect proteins struggled due to the structural instability of proteins, which are sensitive to degradation by proteases [12,18]. Proteins cannot be amplified, similar to the nucleic acids via the polymerase chain reaction. Thus, analyzing small amounts of proteins was challenging and a large amount of proteins per sample was needed for quality assurance and successful proteomic analysis [12]. However, since mass spectrometry (MS) has been established as the modern technology of choice for proteomics, it has provided researchers with high depth, improved accuracy, and unbiased quality [15,19]. Recent technological improvements have allowed the analysis of large-scale proteomes and improved the speed of analysis with short turnaround times [19]. Such technical advances have succeeded in the detection of almost entire proteomes in clinical as well as research samples [20,21]. Furthermore, the enhanced sensitivity and specificity of mass spectrometry, enabling the measurement of minute amount of proteins, has allowed the consideration of proteomics application into future routine clinical practice [22,23].
BASIC PRINCIPLES OF PROTEOMICS
The general aims of proteomic approaches are as follows: (1) identification of specific proteome groups, (2) analysis (e.g., expression levels) of differentially expressed protein signatures from two or more samples, (3) bioinformatic analysis, including the study of protein-protein interactions and gene set enrichment, and (4) study of post-translational modifications in a variety of samples including cell lines, tissue biopsies, and cytology [24,25]. There are two types of proteomic approaches based on the analytic platform used, the protein microarrays and MS-based techniques [26-28]. Regarding the former, there are three types of protein arrays: the analytic microarrays, functional microarrays, and reverse-phase protein microarrays [29]. These arrays have been used to detect differentially expressed protein landscapes, identifying the presence of altered proteins or molecular interactions in certain diseases [30]. However, the restricted number of suitable antibodies needed for such analysis, which could also result in non-specific antigen-antibody interactions, is considered as their main limitation for its use in research or the clinical laboratories [18,28].
During the last years, MS has been significantly improved and emerged as the next generation technology of proteomics, due to its capacity to analyze large-scale proteomes with high sensitivity and specificity [19]. This advanced technique has made protein sequencing possible through three major steps; protein ionization, separation of the ionized analytes based on their own m/z (mass-to-charge) ratio, and detection of the analytes. Finally, the mass spectrum displays the relative abundance of charged analytes vs. their m/z ratios [31,32]. Due to the aforementioned highly accurate and unbiased proteomic analysis through MS, a recent typical proteomic workflow is a mass spectrometry-based one.
THE HISTORY OF PROTEOMIC APPLICATION IN CYTOLOGY
Since the 2000s, numerous studies have utilized high-throughput proteomics in cytology, most of which have been conducted on breast and thyroid specimens (Table 1). In the early days, the two-dimensional gel electrophoresis (2D-GE) was being used for proteomics analysis [33,34], yet this lacked the reproducibility and accuracy of the newer proteomic applications [18]. In this technique, the proteins are initially separated based on their charge and molecular weight with gel electrophoresis. Subsequently, the areas containing the target proteins are excised from the gel and then identified with MS [35]. Through the matrix-assisted laser desorption/ionization time-of-flight mass spectrometry (MALDI-TOF-MS), the cytologic samples are mixed with the substrates, followed by their crystallization within the matrix on a metal plate. Then, the laser energy is absorbed in the matrix generating analyte ions, which are then accelerated into a mass spectrometer [36,37]. In the surface-enhanced laser desorption/ionization timeof-flight mass spectrometry, which is considered as an extended technique of the MALDI-TOF-MS method, the ionized proteins can be directly identified in an electric field by mass spectrometry, without involving protein separation on a 2D gel [38,39]. Over the last decade, electrospray ionization tandem mass spectrometry analysis has become one of the most advanced analytical proteomics methods [40] and has also been applied in cytologic specimens [41].
Regarding breast cancer, most published cytology-based proteomics studies utilized nipple aspirate fluid (NAF), whereas a smaller number FNA samples (Table 1). A few reported significant proteomic profile differences between the NAF of patients with breast cancer compared to non-malignant controls [39,42-44]. In a breast FNA-based study performed by Franzen et al. [45], expression levels of several immune-related proteins differed between cancer and controls, while a few were associated with estrogen receptor, Ki-67 status, and tumor grading. Of interest, liquid-based cytology samples, stored in the methanol-based PreservCyt, were suitable for satisfactory and reproducible proteomic analysis [46], whereas the reverse-phase protein microarrays technology was also applied successfully in breast FNA-based material [47].
To complement the morphologic evaluation of FNA in the evaluation of thyroid lesions, especially the ones with indeterminate interpretations, a few studies utilized in situ proteomics, more specifically the MALDI–mass spectrometry imaging (MSI) technique [48-53]. For instance, MALDI-MSI distinguished benign thyroid lesions from papillary thyroid carcinomas (PTCs) and correctly triaged indeterminate FNA lesions as either benign or malignant [51], while it also distinguished Hashimoto thyroiditis from hyperplastic nodules and PTC in another study [50]. Notably, except differentiating between non-neoplastic lesions from PTC, MALDI-MSI was also able to identify PTC cases carrying the BRAF V600E mutation [49]. Furthermore, Schwamborn et al. applied MALDI-MSI aiming to facilitate Papanicolaou (Pap) test and serous effusion cytologic diagnoses; in situ proteomics was able to correctly assign most lesions into their original cervical cytology classification group and differentiate among diverse cancer types in serous effusions, respectively [54,55].
Apart from breast and thyroid cytology, high-throughput proteomics have additionally been applied in urine cytology, Pap tests, serous effusions, pancreatobiliary samples, salivary FNAs, and bone marrow aspirates (Table 1) with the goal to either improve morphologic diagnosis or identify novel cancer biomarkers. Diagnostic dilemmas in cytology subjected to proteomic analysis have been the differentiation between benign and malignant serous effusions [56,57], pancreatic cancer from autoimmune pancreatitis in FNAs of solid pancreatic lesions [58], inflammatory pancreatic cysts from branch duct intraductal papillary mucinous neoplasms while evaluating cystic pancreatic lesions (BD-IPMNs) [59], non-neoplastic from malignant biliary strictures [60], and benign from malignant salivary gland FNAs [61].
BIOMARKERS DISCOVERED USING CYTOLOGY SPECIMENS THROUGH HIGH-THROUGHPUT PROTEOMICS
Fig. 1 gives a general proteomic workflow used to discover a successful cancer biomarker with cytologic specimens. With the recent advances of MS-based proteomics, even small protein amounts are detectable, while the discovery of biomarker candidates via proteomics has been presented in several studies using cytologic material (Table 2).
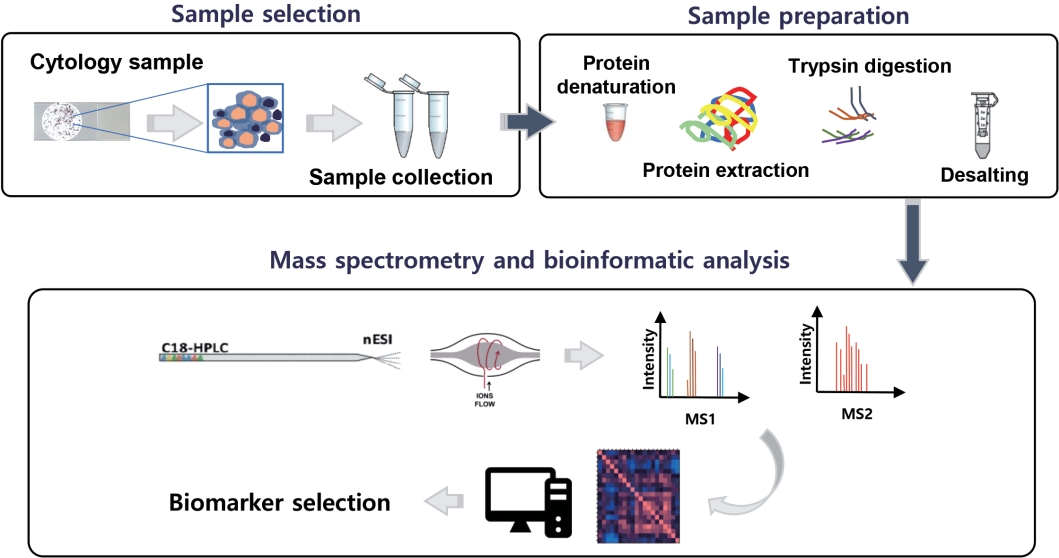
Example of a proteomic analysis workflow utilizing cytology specimens. HPLC, high-performance liquid chromatography; ESI, electrospray ionization.
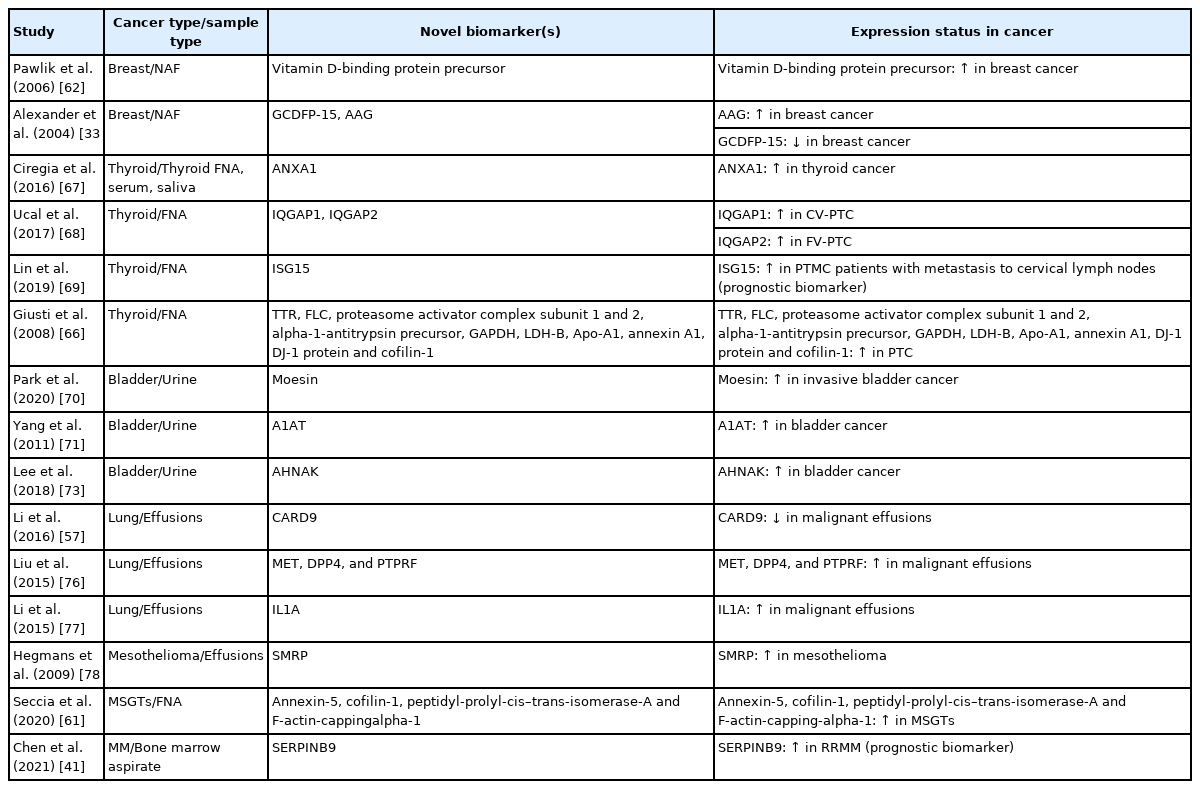
Examples of novel cancer biomarkers discovered by utilizing high-throughput proteomics on cytology samples
Regarding breast cancer, NAF has mainly been used to identify potential breast cancer biomarkers, besides suggesting several proteomic profiles that might have value in assessing the risk of breast cancer (Tables 1, 2). Alexander et al. [33] identified 41 different proteins through 2D-GE and MALDI-MS and suggested two candidate biomarkers, gross cystic disease fluid protein (GCDFP)-15 and alpha1-acid glycoprotein (AAG), testing 52 NAFs from breast cancer patients (in situ and invasive) and 53 controls. GCDFP-15 was found significantly underexpressed, whereas AAG overexpressed in the breast cancer samples [33]. In another study, Pawlik et al. [62] reported that vitamin D binding protein precursor was overexpressed in the NAF of patients with early-stage breast cancer compared to controls.
Thyroid FNAs have often been the subject of proteomics investigation with the goal to solve common diagnostic problems of thyroid cytopathology, for instance the presence of indeterminate thyroid nodules, avoiding unnecessary surgeries (Tables 1, 2). In general, three types of proteomics-based studies using thyroid FNAs have so far been published, aiming to (1) distinguish thyroid cancer from other thyroid lesions [51,53,67], (2) predict lymph node metastasis [69], and (3) predict different PTC variants, currently identified by their histologic characteristics only [66,79]. For example, in a study by Giusti et al. [66], the protein profiles of PTC included several upregulated proteins including transthyretin, ferritin light chain, proteasome activator complex subunit 1 and 2, alpha-1-antitrypsin precursor, glyceraldehyde-3-phosphate dehydrogenase, lactate dehydrogenase chain B, apolipoprotein A1 precursor, annexin A1, DJ-1 protein, and cofilin-1. Ucal et al. [68] reported that several actin cytoskeleton proteins (e.g., Arp 2/3 complex overexpression) were altered in PTC, while IQ motif containing GTPase activating protein 1 (IQGAP1) was upregulated in the classic and IQGAP2 in the follicular variant of PTC, at significant levels, respectively. Torres-Cabala et al. [80] also identified a few thyroid cancer-specific spots using 2D-GE and validated their findings by performing immunocytochemistry on thyroid FNAs, identifying galectin-1, galectin-3, S100C, and voltage-dependent anion channel 1 as candidate tumor biomarkers. Notably, authors in another study—utilizing quantitative proteomics with the quest to identify biomarkers predicting lymph node metastasis—identified 3,793 protein groups, while the interferon-stimulated gene 15 protein was finally selected as a potential biomarker related to lymph node metastasis. Authors also suggested that differentially expressed proteins obtained from cytology samples could be important datasets for the development of new biomarkers [69].
Along with FNA cytology, there have been a few published studies where high-throughput proteomics were utilized on exfoliative cytologic specimens, such as Pap tests [74], serous effusions [57,76,77], bile [60], and urine cytology [70,73]. Boylan et al. [74] showed the residual liquid-based Pap test cytology fixative (SurePath) is a suitable source of protein for MS-based proteomics, reporting the proteome of normal cervical cytology, which was composed of 153 proteins. Regarding serous effusions, caspase recruitment domain family member 9 was found downregulated in malignant effusions [57], overexpression of MET, dipeptidyl peptidase-4, and protein tyrosine phosphatase receptor type F identified metastatic lung adenocarcinomas [76], interleukin 1A was overexpressed in non–small cell lung cancer compared to tuberculosis effusions [77], and serum soluble mesothelin-related protein was identified as a diagnostic biomarker of mesothelioma in pleural effusions [78]. Notably, hepatocyte growth factor and granulocyte-macrophage colony-stimulating factor differentiated inflammatory cysts from BD-IPMNs [59], whereas the overexpression of four proteins (annexin-5, cofilin-1, peptidyl-prolylcis–trans-isomerase-A, and F-actin-capping-alpha-1) differentiated malignant from benign salivary gland FNAs [61].
In two recent studies, our group applied MS-based proteomics on liquid-based urine cytology specimens obtained from urothelial carcinoma patients, and reported potential diagnostic and predictive biomarkers through several validation test layers. The latter included cross validation with TCGA, tumor cell lines with gene editing techniques, and immunocytochemistry in independent patient cohorts [70,73]. Lee et al. [73] selected 112 differentially expressed proteins altered in urothelial carcinoma and validated neuroblast differentiation-associated protein AHNAK (AHNAK) as a new cancer biomarker, able to differentiate between urothelial carcinoma and benign urothelial cytology. TCGA also identified AHNAK as a candidate biomarker along with EPPK1, MYH14, and OLFM4. Furthermore, Park et al. [70] found moesin (MSN) as a potential biomarker predicting the presence of invasive urothelial carcinoma in urine cytology. Of interest, MSN knockdown using siRNA led to inhibition of tumor invasion in urothelial carcinoma cell lines. Also, immunocytochemistry consistently confirmed that MSN is a crucial biomarker predicting invasion when applied in urine cytology [70].
PERSPECTIVES
High-throughput proteomic applications have recently advanced, enabling the use of minimal patient-derived specimens and overcoming the issue of low depth, inconsistency, and suboptimal accuracy. These technical advances are applicable to cytology samples, especially the ones processed with liquid-based cytology, providing reproducible results and revealing a few candidate biomarkers of diagnostic, prognostic, and therapeutic value (Table 2). Most published studies have utilized breast and thyroid cytology samples, showing the potential to help pathologists solve various diagnostic dilemmas and avoid common pitfalls. Such dilemmas comprise the evaluation of indeterminate thyroid nodules while examining thyroid FNAs, the detection of malignant serous effusions, also the differential diagnosis of a few entities in the challenging field of pancreatobiliary cytology, including pancreatic cancer from autoimmune pancreatitis, non-neoplastic from neoplastic pancreatic cysts, and non-neoplastic from malignant biliary strictures. Proteomic profiling of NAF breast samples may identify early-stage breast cancers, also differentiate between in situ and invasive breast cancers and provide information related to prognosis and therapy. Notably, according to the literature, in situ proteomics has exhibited the capacity to triage indeterminate thyroid FNAs thus prevent unnecessary surgeries and reduce healthcare costs, besides provide prognostic information through identifying PTCs carrying the BRAF V600E mutation and predicting the presence of lymph node metastasis or PTC histology associated with a more aggressive behavior (e.g., the tall cell variant) (Table 1). Indeed, proteomic profiling could complement traditional morphologic evaluation and ancillary testing used to examine various exfoliative and FNA cytopathology samples in routine practice or even constitute a stand-alone diagnostic modality in specific settings. However, evidence is still primitive, mostly resulting from studies with small sample size. Apart from the shortage of high-quality evidence, the demands of highly-skilled laboratory personnel, also the cost of analytic equipment, have prohibited the routine application of such approaches and limited them in the research setting. To implement high-throughput proteomics into everyday clinical practice, well-designed prospective studies and randomized controlled trials involving large patient cohorts should be used, aiming to evaluate the proteomics benefits and limitations compared to already established cytomorphologic and ancillary approaches, also their potential implementation in diagnostic algorithms used in cytopathology. Most importantly, cytopathologists and researchers should validate these methods in different sample preparations, and assess their clinical utility in diverse diagnostic scenarios. In conclusion, proteomics could become another diagnostic platform—along with genomics, transcriptomics and/or metabolomics—in the near future, potentially by using validated multi-omics approaches.
Notes
Ethics Statement
Not applicable.
Availability of Data and Material
Data sharing not applicable to this article as no datasets were generated or analyzed during the study.
Code Availability
Not applicable.
Author contributions
Conceptualization: HSR. Project administration: HSR. Supervision: HSR. Writing—original draft: IPN, HSR. Writing—review & editing: IPN, HSR. Approval of final manuscript: all authors.
Conflicts of Interest
The authors declare that they have no potential conflicts of interest.
Funding Statement
No funding to declare.